Tampa Bay
Managing a Public Supply Wellfield Subject to Groundwater Elevation Constraints with an AI Prediction Model
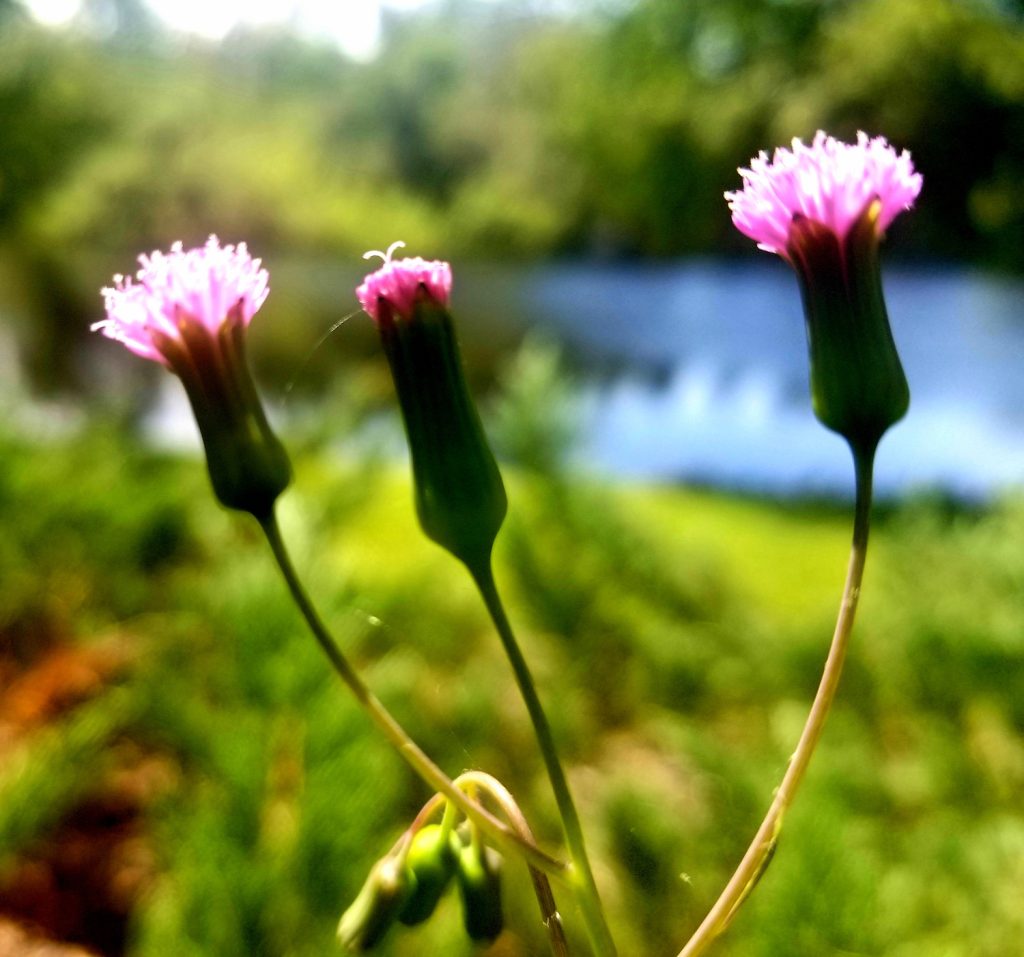
Overexploitation and the resulting aquifer overdraft via unsustainable groundwater extractions is a serious global problem. Affecting nations large and small, in addition to producing adverse environmental impacts, depleting a groundwater system often has devastating economic and social costs, at times posing a regionally existential crisis.
There are far too many cases of severe aquifer overdraft around the world to list here. A few notable examples include the fertile San Joaquin Valley in California, known as the “Breadbasket of the World”, which has destroyed aquatic habitats and caused major land subsidence; the legendary Minqin Oasis, a once lush agricultural region in Northwest China that has become desertified and is now one of the largest sources of dust storms in Asia; and Venice, Italy, which induced significant land subsidence that has exacerbated flooding of this historically and culturally unique ancient city.
The modeling objective for this project, the first of its kind in the world, was to develop AI models with real-world data to accurately predict highly dynamic groundwater elevations in a groundwater system in response to variable pumping and weather conditions.
Tampa Bay, Florida, a booming metropolitan area with one of the fastest growing populations in the United States, is a well-known example of this. As profiled in the bestselling book “Water Follies”, high groundwater extractions of the groundwater system in this densely populated area have produced severe aquifer overdraft, resulting in adverse impacts, including wetlands dewatering, streamflow depletion, and land subsidence. The water utility which operates a high-capacity wellfield is under strict regulatory requirements to prevent groundwater elevations within the unconfined aquifer from declining below imposed target levels. These imposed target groundwater elevations are intended to prevent the above-mentioned environmental destruction of habitats. Failure by the utility to maintain groundwater elevations above these targets can result in steep regulatory fines.
At great financial cost, the water utility developed an extensively calibrated numerical groundwater flow and surface water simulation model, linked to an optimization management model. Using two-week management periods, the utility used this linked system simulator and management optimization model to compute optimal pumping rates for their wellfield that maximizes its total combined groundwater withdrawal without violating target water level constraints.
As depicted by Figure 1, the production wells extract their water from a deeper semi-confined karst limestone aquifer. Above this complex network of fractures and solution cavities is a semi-confined clay layer which separates the limestone aquifer from the unconfined sedimentary aquifer, consisting of a combination of sand with interbedded clay.
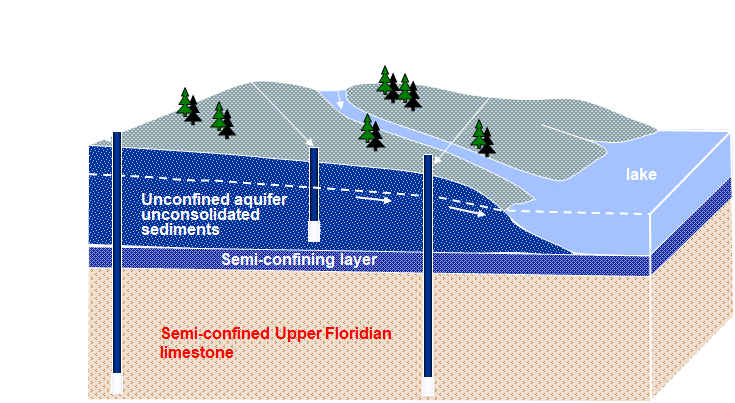
The extreme complexity of the hydrogeologic system in Tampa Bay makes it virtually impossible for even the most advanced physical-based model to accurately predict dynamic groundwater elevations at specific locations in response to variable pumping and weather conditions. Standard Darcian-flow based groundwater flow models like MODLFOW are based on a laminar flow assumption, which karst limestone systems typically violate. Furthermore, attempting to accurately assign a large number of physical model parameter values like recharge, the value of which dramatically varies over space and time in a highly complex multi-layered heterogenous aquifer system, is unrealistic. Lastly, as the numerical model is calibrated to fixed model conditions, unlike AI, the model cannot be reinitialized to real-time conditions, thereby further introducing additional inherent error to any predictions.
STUDY APPROACH AND RESULTS
For this project, AI prediction models were developed using historical groundwater elevations measured at twelve monitoring wells, extraction rates from seven high-capacity production wells, and weather conditions from a nearby airport. Although the number of data events for model development and validation was relatively limited, using two week forecast periods, the AI model accurately predicted highly dynamic groundwater elevations in both the unconfined sedimentary aquifer and the deeper karst limestone aquifer. The AI model not only reproduced dramatic changes in groundwater elevations for two-week ahead prediction periods, but it also significantly outperformed the extensively calibrated numerical model developed by the utility.
To demonstrate the superior AI predictions, its groundwater elevation predictions at the twelve monitoring wells were compared against the utility’s numerical model over a seventy-one-day validation period, consisting of ten consecutive seven-day stress periods. The absolute mean error between the AI predicted and the measured head at the twelve monitoring wells over this 71-day period is just 0.54 feet, compared to the 2.80 feet absolute mean error achieved with the numerical model for the same period.
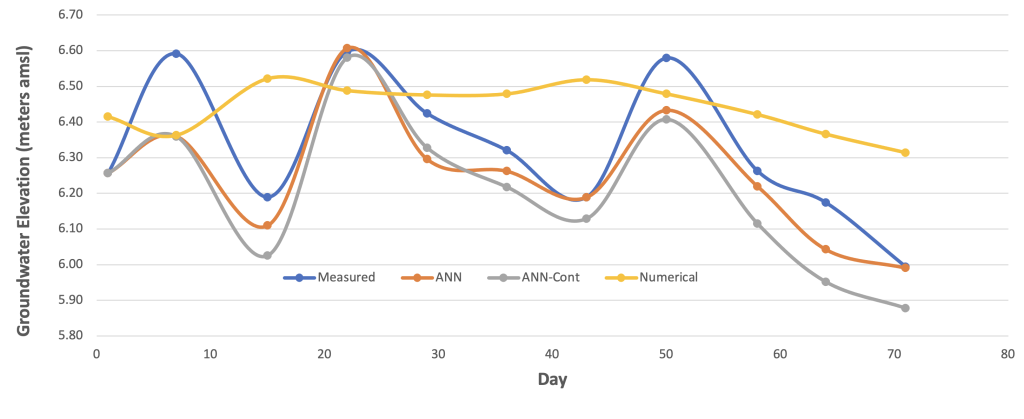
Figure 2. Water levels for validation period in representative unconfined aquifer monitoring well; water level measured in field, predicted by ANN reinitialized with measured data, predicted by ANN reinitialized with its previous prediction (ANN-Cont), predicted by numerical groundwater flow model developed by utility.
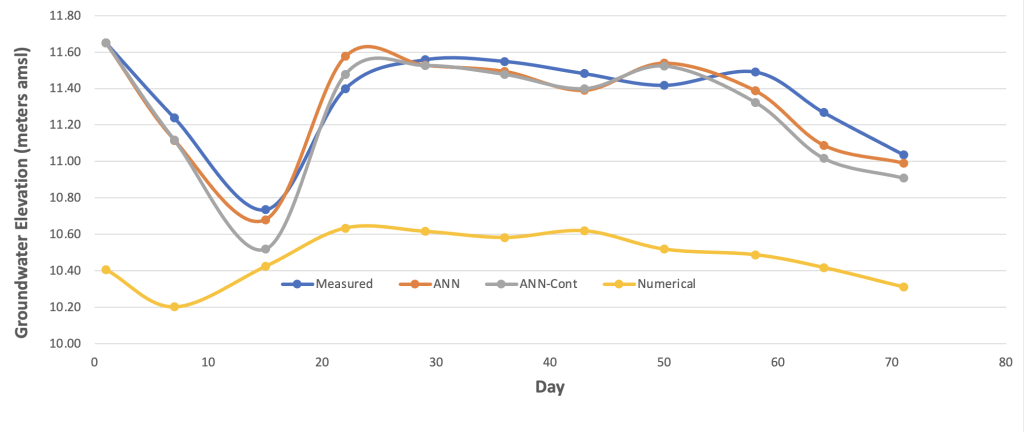
Figure 3. Water levels for validation period in representative semi-confined aquifer monitoring well; water level measured in field, predicted by ANN reinitialized with measured data, predicted by ANN reinitialized with its previous prediction (ANN-Cont), predicted by numerical groundwater flow model developed by utility.
Unlike the numerical model, the AI model accurately predicted the dynamic head responses to pumping and recharge in the complicated multi-layered groundwater system for each two-week ahead prediction period. Even more impressive, the AI model was able to accurately reproduce groundwater elevations in both aquifers over an extended 71-day validation period using ten consecutive seven-day stress periods. For each proceeding stress period prediction, the AI model was re-initialized with its predicted groundwater elevations from the previous stress period.
Figures 2 and 3 compare measured groundwater elevations (i.e., head) versus the utility’s numerical model predictions and the AI predictions for two representative monitoring wells in the unconfined and semi-confined aquifers, respectively. The “ANN-Rei” time series depicts AI groundwater elevation predictions in which the AI model was initialized with the actual measured groundwater elevations at the beginning of the two-week stress period, effectively making it a two-week ahead prediction. The “ANN-Con” time series depicts the AI predicted groundwater elevations in which the AI model was continuously reinitialized with its own predicted values. That is, this AI model simulated the entire 71-day period using its own predicted values as input for the initial conditions for each subsequent prediction. The “Numerical” time series depicts the groundwater elevations predicted by the utility’s numerical model.
As the figures clearly show, there is minimal degradation for the continuous AI model simulation, with a mean absolute error of just 0.59 feet, still dramatically superior to the numerical modeling.
As depicted by Figures 4 and 5, sensitivity analysis was also performed with AI to identify the relative importance of different variables on groundwater elevations in the unconfined and semi-confined aquifers. Unlike traditional physical-based models, where model parameter compensation and non-unique solutions can render sensitivity analysis questionable, the AI models can clearly show direct cause and effect relationships.
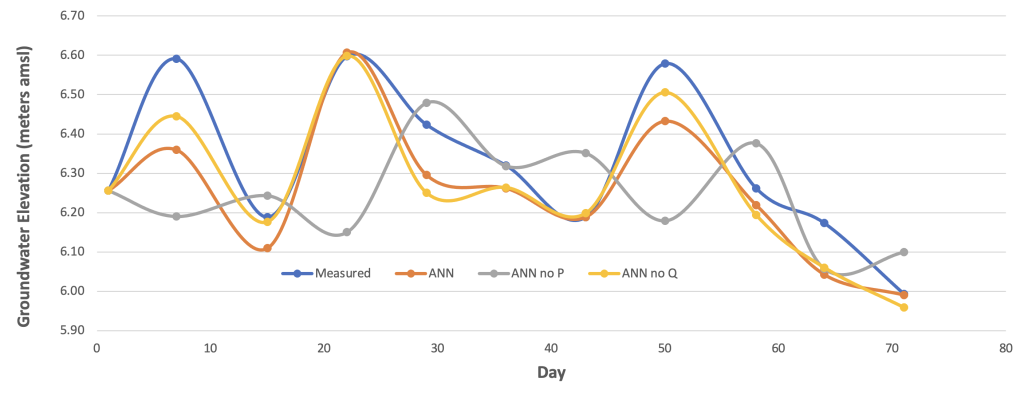
Figure 4. Sensitivity analysis for representative unconfined aquifer monitoring well; water level measured in field, predicted by ANN, predicted by ANN with no precipitation data (ANN-No P), predicted by ANN with no pumping extraction variables (ANN-No Q).
The AI sensitivity analysis demonstrated that over the relatively short two-week optimization periods, groundwater elevations in the shallow surficial aquifer are almost entirely affected by precipitation (P), while groundwater elevations in the deeper limestone aquifer are almost entirely affected by pumping (Q) of the municipal supply wells. These insights into critical cause and effect relationships can provide invaluable water resources management benefits. They include a more complete understanding of the system behavior, improved data collection strategies, improved models with increased prediction capability, an increased awareness of system vulnerabilities and risks, and a more fully informed and rational strategy for regulating and managing the resource.
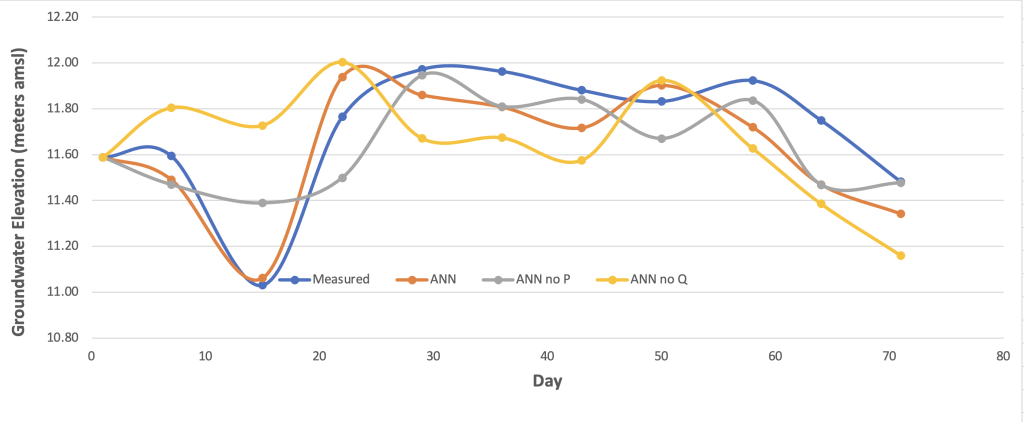
Figure 5. Sensitivity analysis for representative semi-confined aquifer monitoring well; water level measured in field, predicted by ANN, predicted by ANN with no precipitation data (ANN-No P), predicted by ANN with no pumping extraction variables (ANN-No Q).
For example, based on the sensitivity analysis, groundwater extraction rates clearly have nominal effect on groundwater elevations in the shallow aquifer over shorter two-week time periods, whereas weather variables are far more dominant factors. On this basis, then, the regulatory agency might consider modifying the water level targets to also account for weather conditions beyond the control of the utility. Related to this, the utility may increase spatial characterization of weather to improve their AI prediction capability and minimize pumping in areas where AI shows a more direct hydraulic connection between the deeper limestone aquifer and the shallow unconfined aquifer.
The first of its kind, this groundbreaking study proved that AI could be trained using real-world pumping and weather data to accurately predict dynamic groundwater elevations in a highly complex multi-layered aquifer system; that it was also performed using multiple stress periods over an extended simulation period made the results even more impressive. Most importantly, it proved the benefit of using AI as a real-time decision support tool for effectively managing complex groundwater systems, as well as supporting related regulatory strategies and decisions.