TOMS RIVER
Optimally Balancing Public Wellfield Production with Wellhead Protection using an AI Prediction and Multi-Objective Optimization Model
A common but challenging groundwater management problem is balancing the conflicting objectives of maximizing groundwater extraction from public supply wells while minimizing their potential to hydraulically capture known groundwater contamination. Contamination of water supplies often poses a serious risk to human health and may result in high financial costs for treatment and/or replacement of the contaminated water supply.
This AI and multi-objective optimization project, the first of its kind in the world for groundwater management, demonstrates the power of using AI and multi-objective optimization for protecting human health while maintaining an adequate water supply.
The Toms River Parkway Wellfield, made famous by the Pulitzer Prize winning book “Toms River”, is an all too familiar tragic story of a community’s contaminated water supply. As shown by Figure 1, the nearby Superfund site Reich Farm, an illegal industrial waste dumping ground, contaminated the community’s water supply aquifer. Ultimately the groundwater contaminant plume migrated from the site and contaminated two municipal supply wells in the Parkway Wellfield, with four nearby supply wells remaining vulnerable to the contamination.
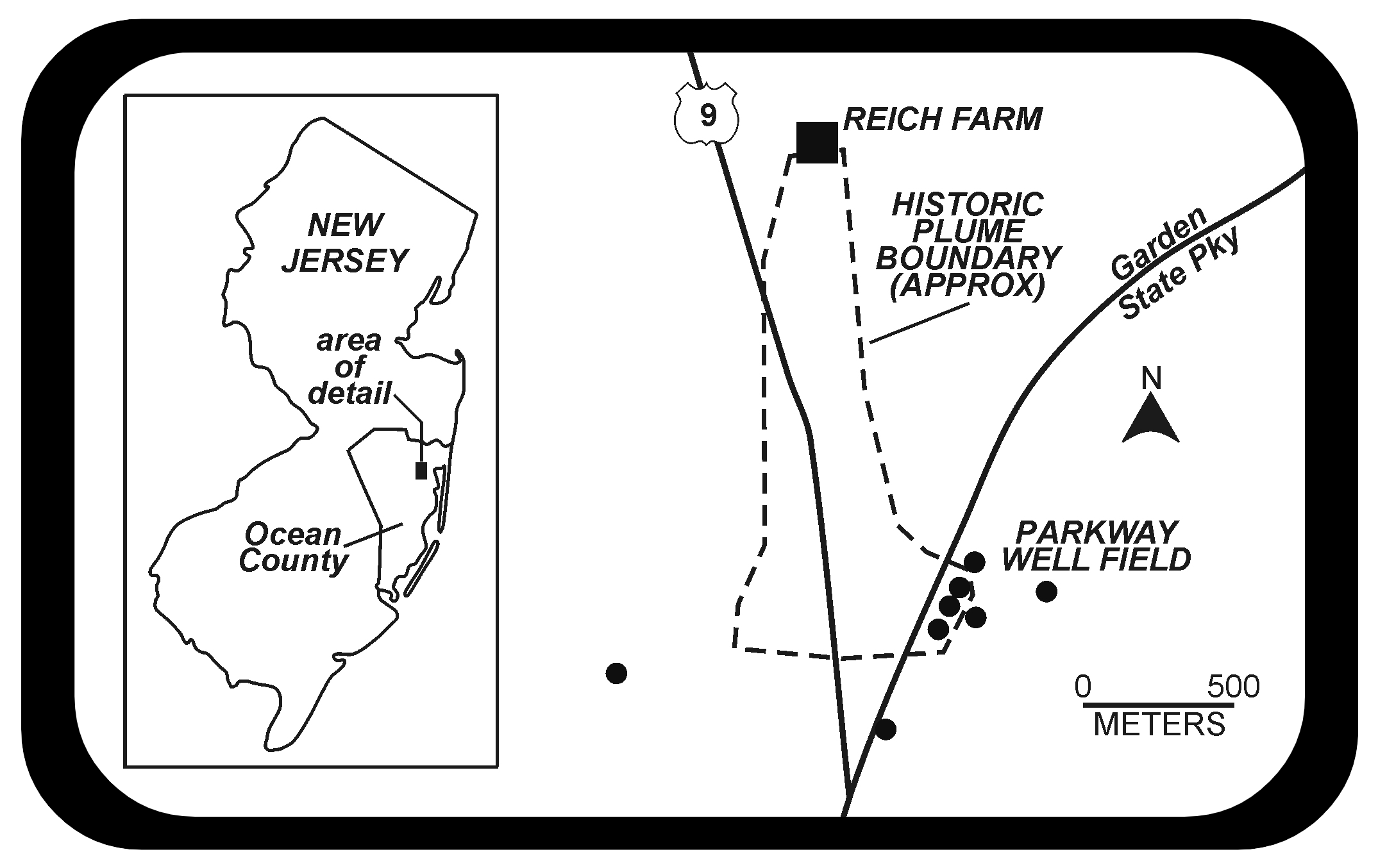
Figure 1. Study Area and Parkway Wellfield
An unprecedented six-year, $10 million-dollar epidemiological study completed on the Township residents and the water distribution system in the early 2000’s concluded that prenatal exposure to contaminated Parkway well water over the years 1982 to 1996 was a risk factor for leukemia in female children living in the community.
Although the Superfund site was remediated, residual groundwater contamination persists near the wellfield at concentrations above safe drinking water standards. The two contaminated supply wells and a third “recovery well” are continuously pumped to prevent the toxic plume from contaminating the nearby “clean wells” used to supply the community with its water. However, during higher demand periods, the clean supply wells must extract groundwater at higher rates, making them hydraulically vulnerable to the plume, potentially exposing the community to contaminants that the water treatment system may not completely remove.
Because of high water demand during summer tourism months, and the availability of only a few alternative water sources, the water utility must balance the objective of meeting the community’s water demand with higher pumping rates while minimizing the wellfield’s vulnerability to the contamination. The problem is further complicated by the intense interests of three main stakeholders: the residents, the water utility, and the chemical company whose waste was dumped without their knowledge at the abandoned farm.
The modeling objectives were to: 1) develop AI models from a numerical groundwater flow model to accurately simulate highly dynamic groundwater elevations around the wellfield in response to variable well extraction rates and weather conditions; 2) combine the AI prediction models with a rigorous formal multi-objective optimization model; 3) generate the non-dominated trade-off curve depicting the wellfield’s vulnerability to groundwater contamination versus its extracted groundwater supply; 4) apply rigorous decision making methods to identify the single best groundwater extraction rates for the supply wells that optimally balance the two conflicting objectives in accordance with the preferences of the stakeholders and the decision maker.
The trade-off curve explicitly defines the optimal trade-off between the total volumetric groundwater extraction of the wellfield and its composite vulnerability to hydraulically capturing the contamination, as measured by relative hydraulic gradients between control pairs. In general, as the extraction wells pump at higher rates, the more vulnerability they are to the groundwater contamination. The difference in water levels between paired locations located on opposite sides of the plume boundary were used to quantify wellfield vulnerability to contamination.
Figure 2 depicts the trade-off curve between the two conflicting objectives over a one-year planning period using monthly pumping schedules for each well. Again, this trade-off curve was generated by integrating the AI groundwater simulation models within a formal multi-objective optimization model that mathematically represented the two conflicting objectives and management constraints.
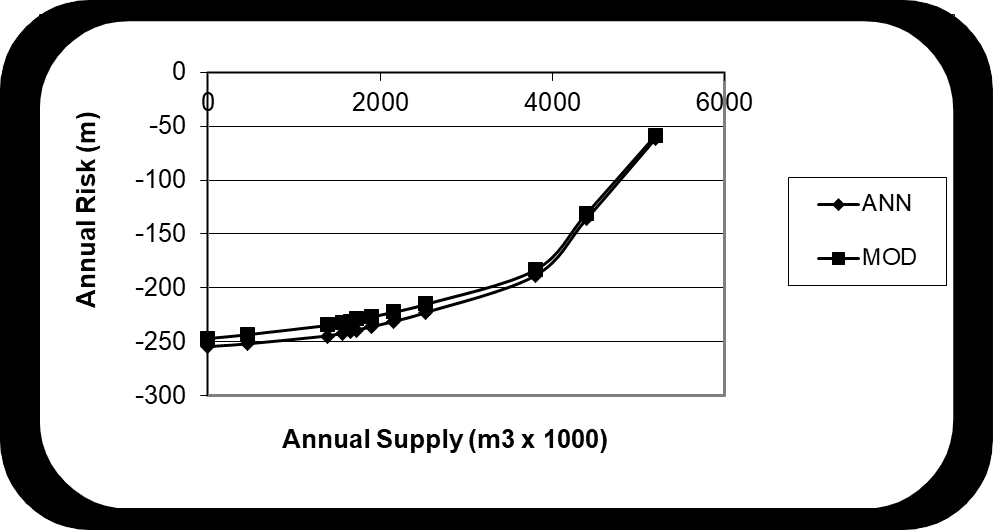
Figure 2. Non-Normalized Pareto Frontiers derived by ANN and verified by MODFLOW (MOD)
Each point or solution along the trade-off curve corresponds to the very best combination of monthly pumping rates for each “clean” supply well that simultaneously maximizes the total annual wellfield extracted groundwater supply while minimizing its total annual vulnerability to contamination for a particular combination of relative weighting or importance values assigned to the two objectives. For example, if zero weight is assigned to the objective of maximizing the total extracted groundwater supply, then the clean wells are all turned off for the entire year. At the other extreme, if zero weight is assigned to the objective of minimizing the wellfield’s vulnerability to contamination, then the clean wells all extract at their maximum rates for the entire year. All solutions between these two extremes represent the optimal relative trade-off between maximizing total groundwater extraction while minimizing wellfield vulnerability to the contamination. Therefore, each solution identifies the optimal distribution of groundwater pumping over both space and time for the two specific relative weight values assigned to the two objectives.
Though rarely performed, this type of rigorous trade-off analysis provides the decision-makers with an explicit delineation of the relative cost and benefit of different operating conditions.
NOAH takes this explicit trade-off analysis to its conclusion by then identifying the single best optimal solution among the infinite number of trade-off solutions or points along the curve. Working with the State regulatory agency, NOAH used various proprietary multi-objective algorithms to identify the optimal wellfield pumping rates in accordance with preferences and priorities of the stakeholders. Under the identified optimal trade-off solution, the water utility can operate the well field at approximately 60 percent of its maximum, while satisfying customer concerns that the existing supply is being protected to the extent practicable.
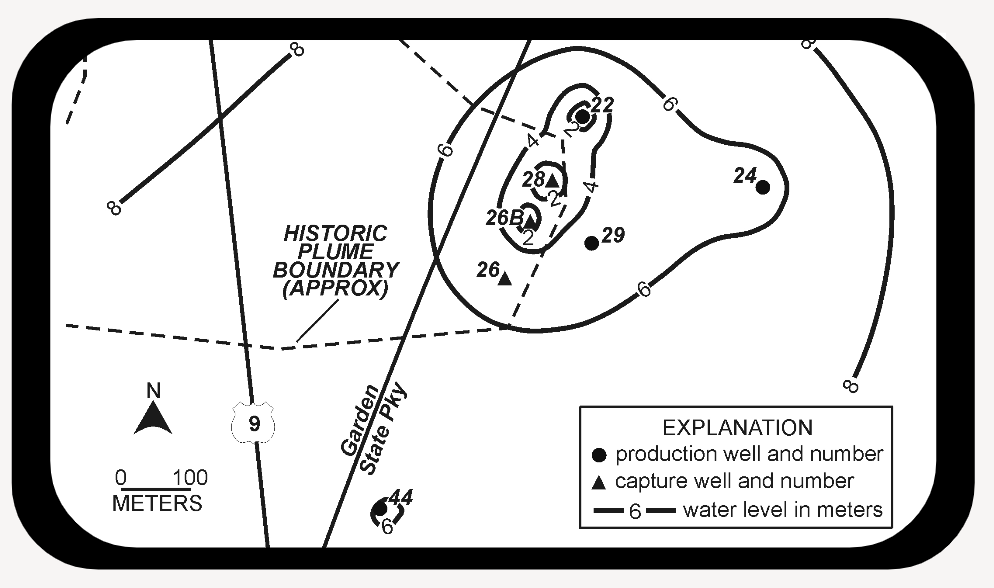
Figure 3. Potentiometric Surface for the Optimal Groundwater Extraction Rates
As shown by Figure 3, the identified solution produced a pumping induced flow field that prevented contamination from reaching the clean wells, while maximizing their potential pumping rates.
That is, the contour lines for the potentiometric surface demonstrate that flow paths of the contaminant plume will clearly converge to the three recovery wells inside the plume, protecting the nearby clean supply wells from contamination. Again, this solution was generated not by first assessing potential contaminant flow paths under different pumping conditions, but by performing the above-described multi-objective analysis. The solution and resulting flow field reflects the somewhat higher preference assigned to protecting the clean wells from the contamination.
The New Jersey Geological Survey concluded that the pumping rates identified by this multi-objective analysis achieved the optimal balance between maximizing total groundwater of the wellfield while minimizing its vulnerability to contamination.
The same methodology can be applied to other problems where pumping needs to be optimized to avoid adverse impacts like saltwater intrusion, wetlands dewatering, and surface water depletion. Similarly, the multi-objective optimization methodology can be applied to virtually any water management problem where the decision maker wants to optimize multiple water management objectives.